Researchers design tools to automatically detect natural disasters using images on social media
A computer vision system based on deep learning has been created that draws on a database containing more than 1.7 million photographsÀgata Lapedriza, who specializes in artificial intelligence for human well-being and is leader of the AIWELL research group, took part in the project during a stay at MIT
"We've demonstrated that it's possible to automatically detect incidents via social media such as Twitter, which could greatly help humanitarian aid organizations"
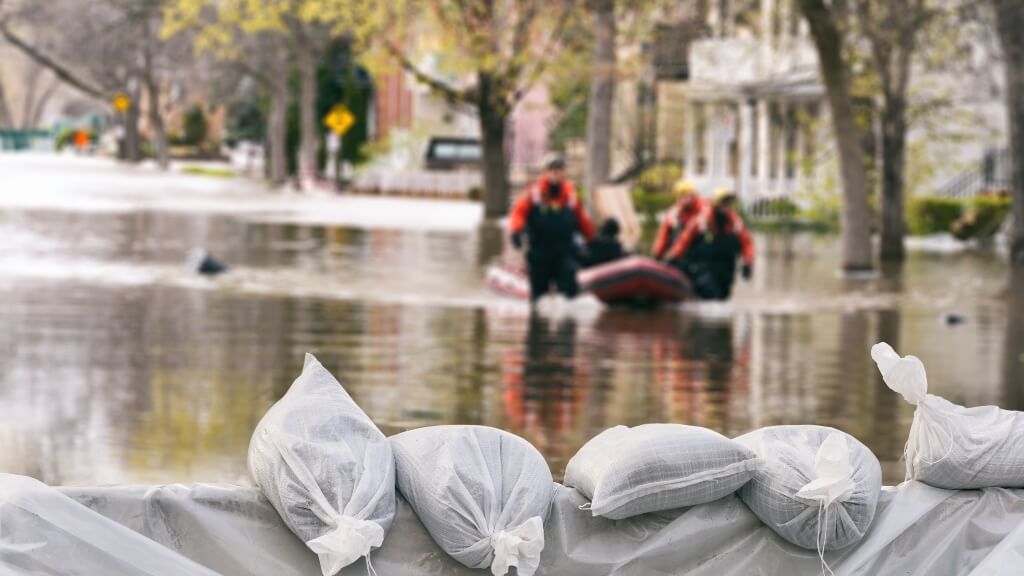
An international research team has designed a deep learning system able to detect natural disasters using images posted on social media. The researchers applied computer vision tools that, once trained using 1.7 million photographs, proved capable of analysing, filtering and detecting real disasters. One of the researchers on the project, led by the Massachusetts Institute of Technology (MIT), was Àgata Lapedriza, leader of the AIWELL research group specialized in artificial intelligence for human well-being, attached to the eHealth Center, and a member of the Faculty of Computer Science, Multimedia and Telecommunications at the Universitat Oberta de Catalunya (UOC).
As global warming progresses, natural disasters such as floods, tornadoes and forest fires are ever more frequent and devastating. As there are still no tools to predict where or when such incidents will occur, it is vital that emergency services and international cooperation agencies can respond quickly and effectively to save lives. "Fortunately, technology can play a key role in these situations. Social media posts can be used as a low-latency data source to understand the progression and aftermath of a disaster," Lapedriza explained.
Previous research focused on analysing text posts, but this research, published in Transactions on Pattern Analysis and Machine Intelligence, went further. During a stay at the MIT Computer Science and Artificial Intelligence Laboratory, Lapedriza contributed to the development of a taxonomy of incidents and the database used to train deep learning models, and performed experiments to validate the technology.
The researchers created a list with 43 categories of incidents, including natural disasters (avalanches, sandstorms, earthquakes, volcanic eruptions, droughts, etc.) as well as accidents involving some element of human intervention (plane crashes, construction accidents, etc.). This list, together with 49 place categories, enabled the researchers to label the images used to train the system.
The authors created a database, named Incidents1M, with 1,787,154 images that were then used to train the incident detection model. From among these images, 977,088 had at least one positive label linking them to one of the incident classifications, while 810,066 had class-negative labels. Meanwhile, for the place categories, 764,124 images had class-positive labels and 1,023,030 were class-negative.
Avoiding false positives
These negative labels meant the system could be trained to eliminate false positives; for example, a photograph of a fireplace does not mean the house is on fire, even though it has some visual similarities. Once the database was constructed, the team trained a model to detect incidents "based on a multi-task learning paradigm and employing a convolutional neural network (CNN)".
When the deep learning model had been trained to detect incidents in images, the team ran a range of experiments to test it, this time using a huge volume of images downloaded from social media, including Flickr and Twitter. "Our model was able to use these images to detect incidents and we checked that they did correspond to specific, recorded incidents, such as the 2015 earthquakes in Nepal and Chile," Lapedriza said.
Using real data, the authors demonstrated the potential of a tool based on deep learning for obtaining information from social media about natural disasters and incidents requiring humanitarian aid. "This will help humanitarian aid organizations to find out what's happening during disasters more effectively and improve the way humanitarian aid is managed when needed," she said.
Following this achievement, the next challenge could be, for example, to use the same images of floods, fires or other incidents to automatically determine the seriousness of incidents or even to monitor them more effectively over time. The authors also suggested that the scientific community could follow up the research by combining the analysis of images with that of the accompanying text, to enable more accurate classification.
This research promotes Sustainable Development Goals (SDG) 3, Good Health and Well-being, and 10, Reduced Inequalities.
Reference article
Weber, E. [Ethan], Papadopoulos, D. [Dim], Lapedriza, À. [Àgata], Ofli, F. [Ferda], Imran, M. [Muhammad] and Torralba, A. [Antonio]. (2023). Incidents1M: a large-scale dataset of images with natural disasters, damage, and incidents. Transactions on Pattern Analysis and Machine Intelligence, 45(4), 4768-4781: https://doi.org/10.1109/TPAMI.2022.3191996
Press contact
Rubén Permuy
rpermuy@uoc.edu
+34 659 05 42 39
UOC R&I
The UOC's research and innovation (R&I) is helping overcome pressing challenges faced by global societies in the 21st century by studying interactions between technology and human & social sciences with a specific focus on the network society, e-learning and e-health.
Over 500 researchers and more than 50 research groups work in the UOC's seven faculties, its eLearning Research programme and its two research centres: the Internet Interdisciplinary Institute (IN3) and the eHealth Center (eHC).
The university also develops online learning innovations at its eLearning Innovation Center (eLinC), as well as UOC community entrepreneurship and knowledge transfer via the Hubbik platform.
Open knowledge and the goals of the United Nations 2030 Agenda for Sustainable Development serve as strategic pillars for the UOC's teaching, research and innovation. More information: research.uoc.edu.
Experts UOC
Press contact
-
Editorial department